Backing up data in the most appropriate way is fundamental for preserving them – and thus avoiding their loss.
There are 3 types of backups that use techniques ranging from simple copies (replication) to back-up systems to archiving.
In the event of a failure, the backup systems will allow the recovery of lost data within a timeframe that is appropriate to the company’s needs.
Archiving allows to keep data that are no longer used but whose conservation can be made mandatory from a legal point of view. It requires a specific process that will be detailed later.
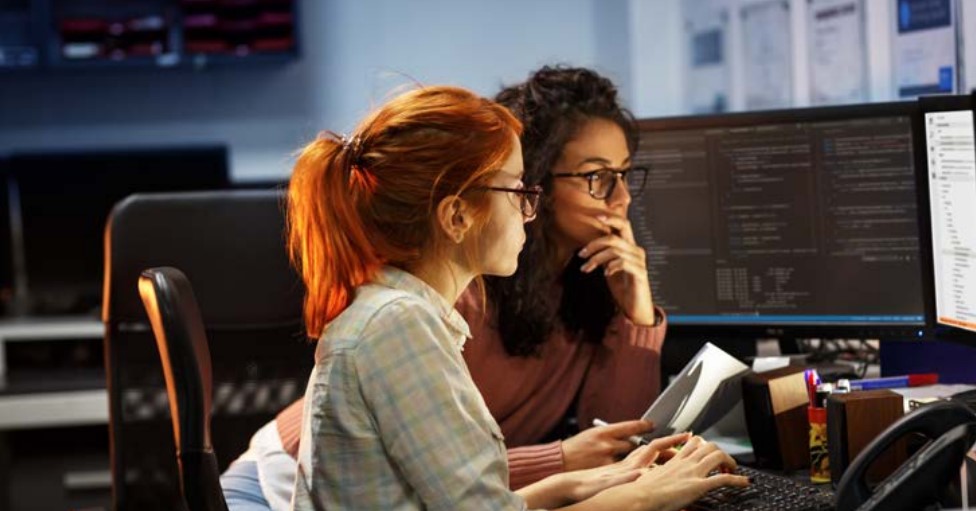
Saving
The copy of a set of data is a preventive process with the objective of securing data. This prevents any eventuality of hardware failure, voluntary or accidental deletion, etc.
Indeed, the process of saving the data from a specific location by copying all files and folders in the system is called a full copy or a full backup.
In some cases, the system stores an additional full copy of the data source while each scheduled copy can be set up on the fifth day of each month. Therefore there will be a full copy of the data from the fifth day of each month.
One of the reasons why some users choose to copy data is that copying is much faster and easier than using a backup tool. However, the process of data copying has some limitations and is often not sufficient. For example, some files in use will not be copied, such as some application configuration files.
Backup
Backup is used for limiting the impact of a potential data loss. The data are copied on a different support – such as an external disk – and data can be restored in case of a data loss.
For that purpose, backup copies are made on secure, durable and regularly tested media.
It is important to note that a continuity or recovery plan to anticipate possible data loss must also be prepared. There are different types of backups, which are described in the next paragraph.
Types of backup
- Full backup: A full backup is a copy of all the data from a specific location. Specifically, when a backup is called a full copy, all files and folders on the system are copied in their entirety and the backup system stores a complete copy of the data source during each scheduled backup.
Example:

- Incremental backup: The incremental backup is the one that is the most used in the context of online backups. It consists in backing up only the data that has been modified or added since the previous full and differential backups. Incremental backup allows focusing only on files that have been modified while using less storage space. In an incremental backup, the initial backup is full and each following backup stores the changes made since the last backup.
Example:

- Differential backup: In a differential backup, the first backup is full. But thereafter, the system only backs up the changes since the last full copy.
Example:

All backup approaches have advantages and disadvantages. Therefore, the data controller will have to determine the appropriate option according to his situation. Once he has chosen the best option, he can determine at which frequency the backup will be performed, for example every 30 rolling days.
Thus it is fundamental to identify prior to the backup, the data that needs to be backed up and also to select the appropriate backup technique according to the organization’s needs.
Saving vs backup
A backup is a copy of data created to restore said data in case of damage or loss. The original data is not deleted after a backup was made.
The definition of backup really comes down to purpose, and the purpose of a backup is always the same: to restore data if something happens to it.
Data backup consists in copying data on an external storage (for example a hard drive, a USB key, a memory card, a cloud, etc.). The copy must contain essential data in redundant form, that is to say in duplicate.
Thus, redundancy is one of the fundamental aspects of a backup, and the main difference with a save. Following a save, data is stored within the system and can be used immediately.
In a nutshell, a backup ultimately allows the data to be totally or partially restored, if old data is needed or if the data is accidentally or intentionally lost.
Versioning
A well-done versioning is a real-time machine that can save you precious time.
Archiving often involves deleting the original version of the data, while backing up involves duplicating the data so that you have multiple versions in the event of a failure on the original version.
Versioning allows you to archive a set of files keeping the chronology of all changes that have been made to them to avoid the risk of corrupting or losing data.
To facilitate versioning, we recommend the implementation of a naming policy allowing rapid identification of minor versions (ex: 1.1. To 1.2.) and major versions (ex: 1.1 to 2.0).
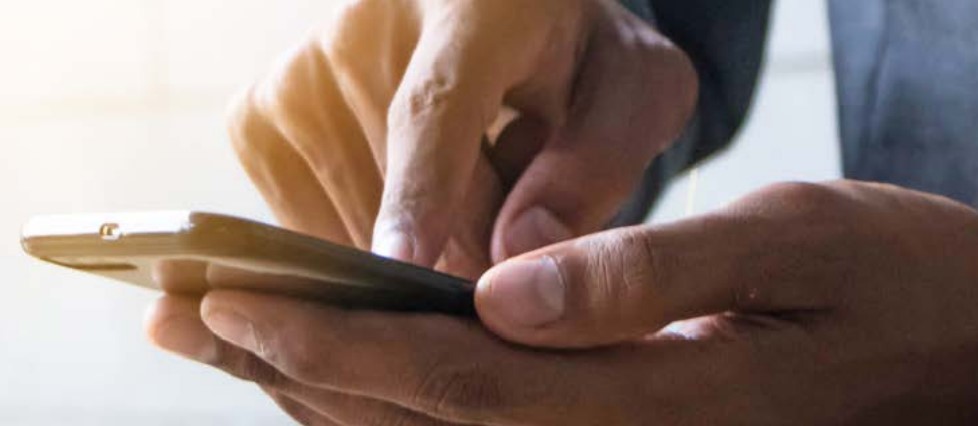
Archive
- What is archiving?
Archiving is the process of retaining data that has ceased to be of current use (inactive) but which needs to be retained, most of the time for regulatory compliance. This process makes it possible to find old data held by an organization and to free up storage space on its information systems. Also, archived data can be used over the long term as evidence in a specific situation (e.g. litigation or regulatory review), which means that archiving must allow the data to be read in the long-term future.
Therefore, the data will have to be archived in a neutral format; this will allow it to be retrieved and read in the future.
- Retention period
Archiving is associated to the duration of data retention stated in the legislation on data protection (GDPR, Data Protection Act).
The data controller will be able to determine the archiving rules from this legislation. Thus, the data controller determines the retention periods of the data he processes in all circumstances, even in the absence of a recommendation from the CNIL or legal regulations. These notions of retention period and archiving are integral parts of the data life cycle.
Archived data can include personal information about an organization’s customers, suppliers, or employees. Therefore, the GDPR applies to protect the privacy of the people involved and the data collected should not be kept for periods that could be considered excessive.
That’s why it is necessary to define the retention period according to the compliance analysis that the data controller must carry out. In some cases, the retention period is set by regulations (for example, article L3243-4 of the French Labor Code requires the employer to keep a duplicate of the employee’s pay slip for 5 years).
However, for many data processing operations, the retention period is not fixed by a text. It is then up to the person in charge ofthe file to determine it according to the purpose of the processing. The data controller must therefore seek the appropriate retention period for the retention of data.
- The different types of archiving
When the data is no longer active, it will either be destroyed or archived.
If the data is archived, then the retention period must be defined. There are two types of archiving:
Intermediary archiving
If the data is no longer of interest for current use but is of administrative interest, for example to provide evidence in the case of litigation, or to meet a legal obligation, it can be archived as “intermediate archiving”.
This is an intermediate step before the data is deleted at the end of the legal period or the limitation period.
However, not all data necessarily need to go through this intermediate archiving phase. Therefore, a detailed case-by case analysis is essential.
At the end of the intermediate archiving phase, the data is either deleted, or permanently archived.
Permanent archiving
Archiving becomes permanent if, at the end of the intermediate archiving period, the data are of “special interest” in the public interest, for scientific or historical research purposes, or for statistical purposes, thus justifying to keep them.
Respect of individuals rights
The data controller must also respect the right to access personal data. In other words, if an individual asks for the data related to him/her that are stored by the organization, the data controller has to send a copy of all these information, whether the data are in an active database or whether they are archived. Also, this copy has to be delivered within a month.
However, the right to erasure or the right to be forgotten does not apply when the processing is necessary to fulfil a legal obligation or in the event of a task of public interest.
Archived Data Protection
Data that no longer need to be processed or used during processing but need to be kept for some reason either legal or for patent or research purposes for
example – will be archived.
- Anonymization
Anonymization is a process that consists in using a set of techniques in such a way as to make it impossible, in practice,
to identify the person by any means whatsoever and in an irreversible manner. Following anonymization, any identification of
a person from a data set must be impossible.
Data anonymization makes it possible to use personal data while respecting the rights and freedoms of individuals.
The technique of anonymization offers multiple advantages. It overcomes the initial regulatory constraints, in that it allows the
exploitation and reuse of the data pool while preserving individual privacy. Finally, the data sets obtained following anonymization
will limit the risks because the data will no longer be deemed to have a personal character.
In addition, the anonymization of data allows for a longer retention period than the initial retention period.
- Pseudonymization
The GDPR defines pseudonymization as the processing of personal data carried out in such a way that data related to a natural person can no longer be attributed without additional information. The additional information must be kept separate and be subject to technical and organizational measures to ensure that it cannot be attributed to identified or identifiable persons.
The pseudonymization technique consists in replacing the directly identifying data (surname, first name, etc.) of a data set with indirectly identifying data (alias, sequential numbers, etc.).
Pseudonymization thus makes it possible to process the data of individuals without being able to identify them directly. The fundamental difference between pseudonymization and anonymization lies in the fact that during pseudonymization, it is often possible to find the identity of the persons involved through third-party data. Thus, pseudonymised data is always deemed to be personal data. In addition, the pseudonymization operation keeps a reversible character, unlike anonymization.
Pseudonymization is therefore one of the measures recommended by the GDPR to limit the risks associated with the processing of personal data. However, the risk to the data is significantly higher in the context of pseudonymization compared to anonymization.
- Encryption
Data encryption is a technique used to convert sensitive or personal information or data that is readable and understandable, into an encoded format to make it unintelligible to users who are not authorized to access it.
Like pseudonymization, encryption is a reversible process: the data is encoded/decoded thanks to a key, which is a decryption algorithm and which will allow to lock and unlock the encryption.
The keys or encryption algorithms are designed to adapt to different uses and are developed when the old ones lose reliability.
In addition to the fact that encryption helps maintaining data integrity, it protects from theft or accidental data loss and helps protecting intellectual property.
- Anonymization techniques
Several techniques exist in order to anonymize data while maintaining the relevance of the dataset, the objective consisting in developing a relevant anonymizing process.
The data controller can thus proceed to:
• Identify the information to be retained according to their relevance;
• Remove direct identification elements as well as rare values that could allow an easy re-identification of persons;
• Distinguish important information from secondary or useless information (i.e. that can be deleted);
• Define the ideal and acceptable level of detail for the information retained.
Anonymization techniques can be grouped into two categories: randomization and generalization.
• Randomization involves changing the attributes in a dataset to make it less precise. This technique aims to alter the link between the individual and the information. For example, it is possible to permute the data relating to the addresses of individuals, in order to affect the veracity of the information contained in a database.
• Generalization, on the other hand, consists in diluting the data or generalizing it by modifying its precision, its scale and its size, in order to ensure that the data set presents characteristics that are common to a set of people. This technique prevents the individualization of a data set, to stay coherent with the way it was written previously and limits its possible correlations with other ones. For example, if a client’s address is specified in Toulouse, this method aims to generalize it to the Haute-Garonne.
Both randomization and generalization must be combined with other techniques to make the anonymization effective.
Attention points:
By using anonymization, the data controller must be aware of certain risks:
• First, the controller must carry out an in-depth assessment of the risk of individuals re-identification, to demonstrate that this risk, using reasonable means, is zero.
• Second, the data controller must regularly monitor technical resources in order to guard against the obsolescence of his anonymization process. The watch must cover both the technical means available as well as the other available sources that could make it possible to lift the data’s anonymity.
After adopting an anonymization technique, the data controller must verify its effectiveness. According to the CNIL and the European data protection authorities, three criteria make it possible to ensure the data anonymization:
• individualization: it should not be possible to isolate an individual in the dataset;
• correlation: it should not be possible to link separate sets of data regarding the same individual;
• inference: it must not be possible to infer, with almost certainty, new information about an individual.
If a technique is able to resist all three of these principles, then it is an effective anonymization technique.
However, it is clarified that to date, no anonymization technique is foolproof. Therefore, particular attention must be paid to
anonymization when it is carried out.