What is Snowflake?
Snowflake is a Data Cloud platform designed to unify, integrate, analyse, and share data at scale and speed. It can process structured, semi-structured, and even unstructured data from various sources, such as databases, files, or streams of data. The Data Cloud is available on Amazon Web Services (AWS), Microsoft Azure, and Google Cloud Platform, in more than 20 regions across the globe.
Since its launch, Snowflake has been dedicated to breaking down data silos by creating a unified and secure place for all data, with a single governance model for all use cases on the platform. Originally focused on analytics, Snowflake has expanded to include data engineering, data science, data-intensive applications, machine learning and artificial intelligence use cases. Snowflake’s goal is to collaborate on all aspects of data, leveraging its incredibly effective compute engine to provide rich and informative experiences to users.
Who is Snowflake for?
Snowflake enables organisations, in particular those situated in an international, complex data environment, or conversely, those that lack IT and DBA resources internally to exploit their data easily and quickly.
As a fully self-managed cloud service, there’s no need to select, install, configure, or manage any hardware, virtual or physical. You can expect virtually no software installation, configuration, or management. Ongoing maintenance, management, upgrades, and tuning are handled by Snowflake. Additionally, Snowflake runs entirely on cloud infrastructure, with all service components (excluding optional command line clients, drivers, and connectors) running in public cloud infrastructures.
What makes Snowflake architecture unique?
Snowflake has a central data repository for persisted data that can be accessed by all compute nodes in the platform. Queries are processed using massively parallel processing (MPP) compute clusters, with each node in the cluster storing a portion of the entire data set locally. This approach provides easy data management, while also delivering exceptional performance and scale-out advantages.
Snowflake’s unique architecture has three layers:
- Database Storage
Snowflake reorganises, optimises and compresses the data loaded into the platform and stores it in a columnar format. Customers can only access the stored data through SQL queries using Snowflake since the data objects are not directly visible or accessible.
- Query Processing
Snowflake processes queries using virtual warehouses, which are MPP compute clusters consisting of multiple nodes allocated from cloud providers. Virtual warehouses are independent and don’t share compute resources, allowing one to operate without affecting the performance of the others.
- Cloud Services
This layer is a collection of services that organise activities across Snowflake, including authentication, access control, query parsing and optimisation, metadata management and infrastructure management.
Unlike traditional data warehouses that rely on static partitioning of large tables, Snowflakes uses a unique micro partition format that delivers performance and scale without known limitations, such as data skew and maintenance overhead. This means that the platform allows concurrent reading and writing of data without locking/blocking, and rapid undos of deletes/inserts/edits by modifying pointers to data blocks. The experience of managing data in Snowflake feels a lot more intuitive, and a lot less risky due to dropping/restoring functionality and possible time travel functionality on all data objects on the platform.
The Time Travel feature is based on Snowflake’s data versioning system, which allows users to query snapshots of data as it existed at a specific point in time, or as it existed up to a certain number of days ago. This feature can be useful for auditing, investigating, and correcting data issues – and the related cloning feature can be used to virtually eliminate the need for pre-production environments for testing data products.
Sharing data with Snowflake
You can share live, ready-to-query data across Snowflake accounts without any data movement, as long as the cloud region is the same. In addition to sharing data, you can also share business logic and services, ensuring your ecosystem has the data and tools it needs. If data sets and apps are put on the Snowflake marketplace or a private exchange, it can even be distributed across clouds and regions, after native and secure replication of the content is in place.
Snowflake offers fine-grained governance and access control to ensure security and compliance with industry and region-specific data regulatory requirements.
How does Snowflake tap into machine learning (ML) and artificial intelligence (AI)?
By consolidating all your data in one location, an ML or AI process becomes significantly easier. Initiating ML or AI algorithms natively on Snowflake, close to where data resides, is enabling businesses to bypass the need for complex data pipelines and additional governance processes, thereby accelerating data operations and time to market for enterprise data products. Through centralised and streamlined operations, organisations can natively bring AI applications to life using Snowflake.
Snowflake has additionally built Snowpark, a developer environment for apps and ML models based on the Anaconda distribution. It covers the languages of Java, Python, and Scala, to implement models and applications easily. Snowpark ML Modeling, currently available as an open preview feature, has Python APIs for preprocessing data and training models.
What are the main benefits of Snowflake?
- Break down barriers of siloed data in your organisation
For decades, siloed data both on-premise and in the cloud have limited insights, creating significant business challenges. Snowflake delivers unlimited scalability and concurrency, unique data-sharing capabilities, and a Data Marketplace for sharing data across departments, subsidiaries, geographies, and with your business partners.
- Reduce query times from hours to seconds
Materialized views (available in the Enterprise Edition) are pre-computed datasets that can provide faster querying than querying against base tables. Materialized views excel when costly operations like aggregation, projection, and selection are frequently run on large datasets.
- Built-in governance
Snowflake Horizon is a unified set of capabilities for compliance, security, interoperability, data access, and privacy within Snowflake’s Data Cloud. It includes features to safeguard your data, maintain business continuity, monitor data quality, and track data lineage.
- Get value from data with AI
Snowflake’s recently launched features make it possible for any user to include LLMs in analytical processes. Developers can build GenAI-based applications and execute powerful workflows like fine-tuning foundation models on enterprise data.
In conclusion
By combining data warehouse, data lake, data engineering, data science, business apps and data sharing services, Snowflake spans the entire space between data sources and end users. Natively multi-cloud, multi-geography, and disarmingly easy, the Snowflake platform breaks down both the organisational and technical barriers of traditional data infrastructures to create a one-stop shop for data and democratises its use in the enterprise. Along with the unique architecture, the newly announced AI features enable analysts, data scientists, developers, and business leaders to unlock the full potential of data.
Want to assess Snowflake’s relevance and potential for your organisation?
Connect with one of our experts today and find out if Snowflake is the right solution for you.
This article and infographic are part of a larger series centred around the technologies and themes found within the 2023 edition of the TechRadar by Devoteam report. To learn more about Snowflake and other technologies you need to know about, please download the TechRadar by Devoteam .
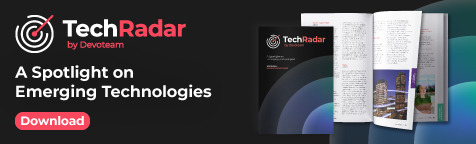